Video Analytics: Detect Anomalies & Boost Manufacturing
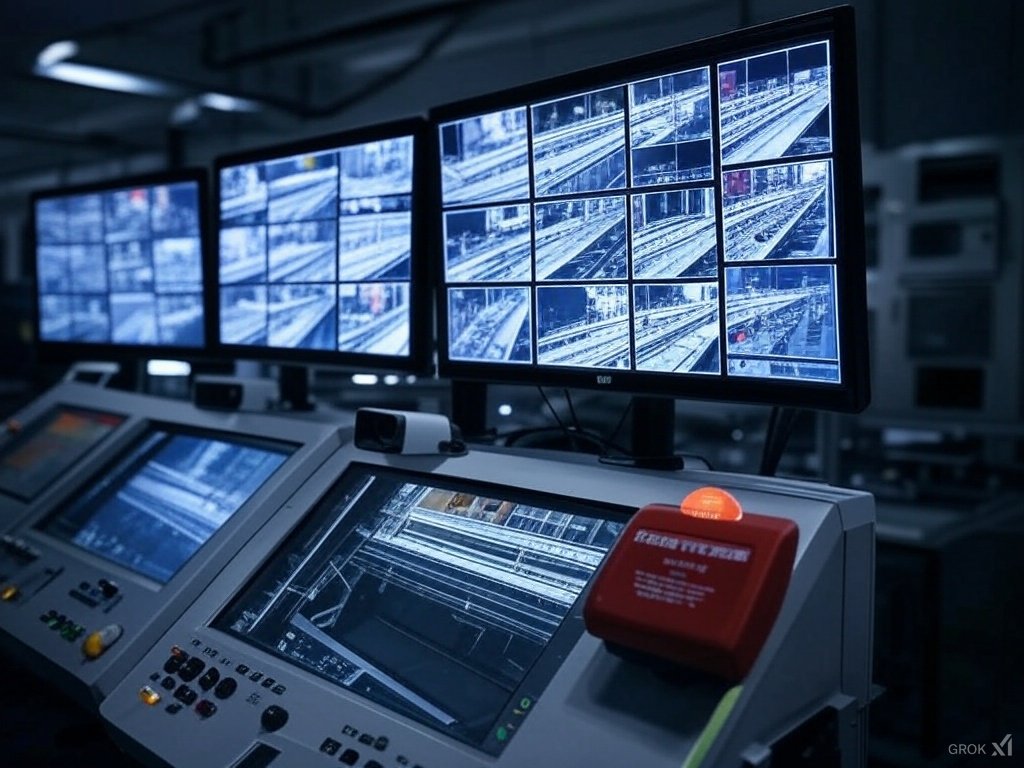
In today's fast-paced manufacturing environment, maintaining seamless operations and ensuring product quality are paramount. Production anomalies, such as glass jams or fallen containers, can disrupt workflows, lead to costly downtime, and compromise product integrity. Traditional methods of anomaly detection often rely on manual inspections or basic sensor data, which can be inefficient, inconsistent, and prone to human error. However, with the advent of advanced video analytics powered by artificial intelligence (AI), a new era of real-time, automated anomaly detection has emerged, promising to revolutionize the manufacturing landscape.
This blog post delves into the transformative power of video analytics in detecting production anomalies. We'll explore how AI-driven systems can continuously monitor production lines, identify deviations from the norm, and trigger automated responses to mitigate disruptions. We'll also examine the benefits of implementing video analytics solutions, including improved efficiency, reduced costs, enhanced quality control, and increased safety. Join us as we uncover how this cutting-edge technology is reshaping the future of manufacturing and paving the way for more resilient and optimized production processes.
Understanding Production Anomalies and Their Impact
Production anomalies encompass a wide range of unexpected events or deviations from the normal operating conditions of a manufacturing process. These anomalies can arise from various sources, including equipment malfunctions, material defects, human errors, and environmental factors. Regardless of their origin, production anomalies can have significant consequences for manufacturers, impacting:
- Product Quality: Anomalies can lead to defects, inconsistencies, and non-conformances in finished products, resulting in customer dissatisfaction and potential recalls.
- Operational Efficiency: Disruptions caused by anomalies can slow down production lines, reduce throughput, and increase cycle times, leading to decreased overall efficiency.
- Cost: Downtime, rework, scrap, and warranty claims associated with anomalies can drive up manufacturing costs and erode profit margins.
- Safety: Certain anomalies, such as equipment failures or hazardous material spills, can pose safety risks to workers and the environment.
Given the potential impact of production anomalies, it's crucial for manufacturers to implement robust detection and response mechanisms. Traditional methods often fall short in providing the real-time visibility and automated intervention needed to effectively address these challenges. This is where video analytics and AI offer a game-changing solution.
The Power of Video Analytics in Anomaly Detection
Video analytics leverages the capabilities of computer vision and machine learning to automatically analyze video streams from cameras strategically positioned along production lines. By continuously monitoring the visual data, these systems can identify patterns, detect anomalies, and trigger alerts in real-time. Here are some key ways in which video analytics enhances anomaly detection:
- Continuous Monitoring: Video analytics systems operate 24/7, providing continuous surveillance of production processes without the limitations of human fatigue or attention lapses.
- Real-Time Detection: Anomalies are identified as they occur, enabling immediate intervention to prevent further escalation or damage.
- Automated Alerts: Upon detecting an anomaly, the system can automatically trigger alerts, such as sending notifications to operators or activating alarms, ensuring timely response.
- Data-Driven Insights: Video analytics systems generate valuable data and insights into the frequency, nature, and causes of anomalies, enabling manufacturers to identify trends and implement preventative measures.
How AI-Driven Video Analytics Works
AI plays a crucial role in enabling advanced video analytics for anomaly detection. Machine learning algorithms are trained on vast datasets of video footage to learn the characteristics of normal and abnormal operating conditions. Once trained, the AI model can automatically identify anomalies based on visual cues, such as:
- Object Recognition: Identifying and tracking specific objects, such as products, containers, or equipment components, to detect missing or misplaced items.
- Motion Analysis: Detecting unusual movements or patterns, such as sudden stops, jerky motions, or unauthorized access.
- Appearance Changes: Identifying changes in the appearance of objects, such as dents, cracks, or spills.
- Spatial Relationships: Analyzing the spatial relationships between objects, such as proximity, alignment, or orientation, to detect deviations from the norm.
The AI model continuously refines its accuracy and performance through ongoing learning and adaptation, ensuring that it remains effective in detecting a wide range of anomalies over time. Furthermore, sophisticated AI models can differentiate between different types of anomalies, allowing for tailored responses based on the severity and nature of the event.
Use Cases: Real-World Examples of Video Analytics in Anomaly Detection
Video analytics is being deployed across a wide range of manufacturing industries to address various anomaly detection challenges. Here are some real-world examples:
- Food and Beverage: Detecting fallen containers on conveyor belts, identifying mislabeled products, and monitoring fill levels in bottles or cans.
- Automotive: Detecting defects in car bodies, identifying missing components in assembly lines, and monitoring worker safety compliance.
- Pharmaceuticals: Ensuring proper packaging and labeling of medications, monitoring cleanliness in sterile environments, and detecting unauthorized access to restricted areas.
- Electronics: Detecting defects in circuit boards, identifying misaligned components, and monitoring temperature fluctuations in sensitive equipment.
In each of these use cases, video analytics provides real-time visibility into production processes, enabling manufacturers to quickly identify and address anomalies, minimizing disruptions and ensuring product quality.
Benefits of Implementing Video Analytics for Anomaly Detection
Implementing video analytics for anomaly detection offers a multitude of benefits for manufacturers, including:
- Improved Efficiency: Real-time detection and automated responses minimize downtime and optimize production flow.
- Reduced Costs: Early detection of anomalies prevents costly rework, scrap, and warranty claims.
- Enhanced Quality Control: Consistent monitoring and identification of defects ensure higher product quality and customer satisfaction.
- Increased Safety: Detection of hazardous conditions or unsafe behaviors promotes a safer working environment.
- Data-Driven Decision Making: Analytics provide valuable insights into production processes, enabling continuous improvement and optimization.
By leveraging the power of video analytics, manufacturers can transform their operations, achieving greater efficiency, quality, and safety.
Key Considerations for Implementing a Video Analytics Solution
When implementing a video analytics solution for anomaly detection, it's important to consider the following factors:
- Camera Placement: Strategically position cameras to capture critical areas and processes.
- Lighting Conditions: Ensure adequate lighting to provide clear and consistent video footage.
- Network Infrastructure: Provide sufficient bandwidth to support real-time video streaming and processing.
- AI Model Training: Train the AI model on a representative dataset to ensure accurate anomaly detection.
- Integration with Existing Systems: Integrate the video analytics system with other manufacturing systems, such as ERP or MES, for seamless data sharing and workflow automation.
- Data Privacy and Security: Implement robust data privacy and security measures to protect sensitive information.
By carefully addressing these considerations, manufacturers can ensure the successful implementation of a video analytics solution that delivers tangible benefits.
The Future of Video Analytics in Manufacturing
The future of video analytics in manufacturing is bright, with ongoing advancements in AI, computer vision, and edge computing paving the way for even more sophisticated and powerful solutions. Some emerging trends include:
- Edge AI: Processing video data directly on edge devices, such as cameras or gateways, reducing latency and bandwidth requirements.
- 3D Vision: Using 3D cameras to capture depth information, enabling more accurate and detailed anomaly detection.
- Predictive Analytics: Leveraging AI to predict potential anomalies before they occur, enabling proactive maintenance and preventative actions.
- Human-Machine Collaboration: Integrating video analytics with human expertise to create a collaborative environment where humans and machines work together to optimize production processes.
As these technologies mature, video analytics will play an increasingly vital role in transforming manufacturing operations, enabling greater efficiency, quality, and resilience.
In conclusion, video analytics powered by AI is revolutionizing anomaly detection in manufacturing. By continuously monitoring production lines, identifying deviations from the norm, and triggering automated responses, these systems are enabling manufacturers to improve efficiency, reduce costs, enhance quality control, and increase safety. As the technology continues to evolve, video analytics will play an even more critical role in shaping the future of manufacturing, paving the way for more resilient, optimized, and intelligent production processes. Embrace the power of video analytics and unlock the full potential of your manufacturing operations.
Ready to explore the possibilities of AI-powered video analytics? Check out the Securade.ai HUB repository on GitHub and give us a star!